How to Handle Tables During Chunking?
- Metric Coders
- Mar 29
- 3 min read
Tables are the hidden gems in documents—packed with structured, high-value information. But when it comes to chunking documents for NLP or LLM pipelines, tables are tricky.
Unlike plain text, tables:
Don’t follow standard sentence structure
Span multiple rows/columns
Often break when extracted naively
So, how do you handle tables effectively during chunking?
Let’s break it down.
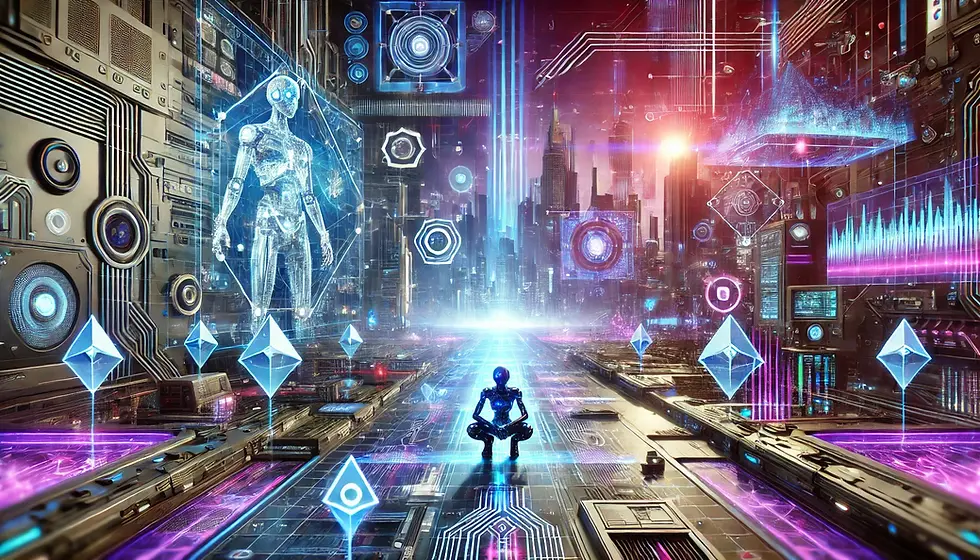
🧩 Why Chunking Tables Is Hard
Imagine you're processing a PDF annual report or research paper:
A table might start mid-page and continue across the next
It may include footnotes, merged cells, or nested headers
A naive chunking strategy might split a table in half 😬
The result? Broken context, meaningless data, and unusable chunks.
✅ The Right Way to Handle Tables in Chunking Pipelines
Here’s a step-by-step method that works reliably across most document pipelines.
🥇 Step 1: Detect Tables Separately
Use a layout-aware parser that can distinguish tables from paragraphs.
Tools that work well:
pdfplumber – Great for detecting and extracting table regions
Unstructured.io – Tags content types like Table, Title, Narrative, etc.
Adobe PDF Extract API – High accuracy for structured tables
camelot / tabula – Ideal for cleanly structured tables in PDFs
🧠 Tip: Treat tables as first-class citizens, not inline text.
✂️ Step 2: Chunk Tables as Atomic Units
Once detected, do not split tables during chunking.
Instead:
Extract the full table block
Convert it to a structured format (like JSON, CSV, or Markdown)
Treat the table as one chunk
{
"chunk_id": "table_12",
"type": "table",
"section": "Financial Summary",
"page": 33,
"table_data": [
["Year", "Revenue", "Profit"],
["2022", "$10M", "$2M"],
["2023", "$12M", "$2.5M"]
]
}
This ensures the model sees the full context, without fragmented rows or headers.
🔎 Step 3: Add Metadata & Context
Tables without context are just data dumps.
Add:
Surrounding text (introductory paragraphs or captions)
Section title (e.g., “Consolidated Financials”)
Table title or number (e.g., “Table 2.1: Revenue Breakdown”)
Combine these into a structured chunk with both table and text context.
{
"type": "table",
"title": "Table 2.1: Revenue Breakdown by Region",
"preceding_text": "The table below shows the year-over-year revenue comparison across key regions.",
"table_data": [...],
"page": 21
}
🧠 Step 4: Choose the Right Format for LLMs
Tables can confuse language models when not formatted properly.
Recommended formats:
Markdown Tables (for GPTs, Claude, etc.)
JSON (for structured parsing or programmatic QA)
Natural language summaries (optional, as fallback)
💡 Use Markdown for LLM summarization or QA, JSON for RAG/vector DB pipelines.
🔁 Step 5: Don’t Overlap Tables With Text Chunks
Avoid mixing part of a table with part of a paragraph chunk.
Keep table chunks separate
Keep narrative chunks clean
Use chunk type labels (table, narrative, header, etc.)
This makes downstream tasks like embedding, vector search, or summarization more accurate and efficient.
🚫 What Not to Do
❌ Splitting tables row-by-row❌ Merging table cells with unrelated paragraphs❌ Ignoring multi-page tables❌ Treating table text like plain prose
All of these result in broken logic and poor AI responses.
📊 Use Case: Table-Aware LLM Querying
Here’s how you might use table chunks in a retrieval-augmented generation (RAG) pipeline:
Embed table chunks (as Markdown or JSON)
Store them in a vector database
Use natural queries like:
“What was the net income in 2022?”“Compare revenue by region between 2022 and 2023”
The LLM now sees clean, contextual tables and can reason accurately.
🧪 Bonus: Generate Summaries for Each Table
Use the LLM to automatically summarize the key insights from each table.
{
"summary": "Revenue increased 20% YoY, driven primarily by North America.",
"source_table": "table_12"
}
Now you’ve made the table both human-readable and machine-usable.
🧠 Final Thoughts
When chunking documents for LLMs, tables need special treatment. If you handle them with care—detect, isolate, format, and annotate—you’ll unlock a goldmine of structured knowledge.
🛠 TL;DR Cheat Sheet:
Task | Recommendation |
Detect tables | Use pdfplumber, Unstructured, Adobe, camelot |
Chunking | Never split tables mid-way |
Format | Use Markdown (for LLMs) or JSON (for structured pipelines) |
Context | Add titles, captions, and surrounding text |
Post-process | Optional summaries for QA or dashboards |